In our last post about the “Twelve Shifts of Digital”, we spoke about the shifts around architecture. This week, we explore the subject of data and the shift from “reactive analytics and federated data, to data-led and insights embedded into journeys and decision making”.
Many organisations still find themselves struggling with a legacy of poor quality data which cannot be connected across silos, inconsistent management information and insights, and are stuck in a death loop of endless PoCs, unable to get anywhere near realising the promise of artificial intelligence. As such, they find themselves relying on HIPPO decision making (Highest Paid Person’s Opinion), drowning in a murky soup of gut instinct, misinformation and hearsay.
Meanwhile, the leading digital organisations - built on a foundation of real-time, integrated and high integrity data, who have placed insight and automation at the heart of their organisation - race off into the sunset, fuelled by deep customer insight, forensic understanding of their operational performance and fully automated, machine-learning powered processes.
We have known for many years that data-driven organisations are both more productive and more profitable than their less enlightened peers (Source: Big Data - The Management Revolution; Harvard Business Review), so harnessing the power of data and insight is no longer just a “nice to have”, it is a matter of survival.
But how can this be achieved? What are the key components of a data and insight-driven organisation? And what does the journey look like to get there?
-------------------------------------------------------
IDEA – Inspiration / Discipline / Embedding / Action
Inspiration: The importance of a Vision & Strategy
A compelling vision, which paints a picture of how data and insight will transform the daily lives of employees, enables richer interactions with customers, and accelerate and automate processes must inspire the organisation and act as a “north star” throughout the transformation journey.
Whilst the vision is required to seduce the right-brain into action, the strategy satisfies the left-brain - providing practical steps for how the organisation will reach its goals. Without both, organisations struggle to break the inertia or end up with a collection of piecemeal, discordant initiatives.
Discipline: Architecture and Governance
The hard yards. This is the proving ground. This is where the dirty, difficult, fastidious, but ultimately most critical work is done. Having clarity on what data means, knowing where data is stored, knowing the people and processes that touch that data, monitoring the quality of data and fixing it when it is wrong, knowing how data can be used, with whom it can be shared, when it has to be deleted, who within the organisation is responsible for it, maintaining a view of the relationships between data that create the information the business requires, ensuring that new projects or change doesn’t compromise all the hard work that has been put in to create a “trusted” data set…and documenting everything.
Keeping that documentation up to date is critical so that people don’t have to go on a wild goose chase every time they need data. It’s boring, but this is what separates the data-driven organisations from the organisations driven crazy by data.
Embedding: Putting data and insight at the heart of everything
People hunting for data and insight in many organisations can often feel like they are re-living the experience of Coleridge’s ancient mariner: “Water, water, everywhere, but not a drop to drink” is translated into “We have loads of data, but no insight”.
The first step is to make data available to all those who need it, when they need it, in a consumable format. Data pipelines must be built, channelling data from source systems to warehouses, and then into reports or machine learning models – with data integrity checks providing assurance along each step of the journey and a “semantic” layer enabling interaction with the data through clearly defined terminology. Organisations should also look beyond their own boundaries to enrich their data with open data sources, and can create “greater than sum of the parts” data sets by collaborating with like-minded organisations to enhance customer insight.
Insights and the automated outputs from machine learning models must also be embedded into processes, to ensure that at all stages, the power of data is being harnessed. There are many organisations that have spent millions creating the infrastructure and teams required to generate insight – but have not changed their ways of working to consult or integrate that insight. A culture which places evidence ahead of gut instinct must be embedded, with the best examples being celebrated and shared across the organisation. It is not enough to “wish” that culture will change, this insight-driven tone must be set from the top – but the tools and data must also be provided to make it easy for the transformation to be driven from the ground up.
In order to move machine learning models beyond the PoC and into production, organisations also need to embed software development discipline and a DevOps approach. Data scientists must be embedded alongside software developers, data engineers, IT operations and of course business stakeholders and design their models with a “production-ready” mindset. Continuous Integration and Continuous Deployment pipelines, built on scalable infrastructure, enable these models to rapidly move from theory to reality.
Action: The Saw Model for Data Transformation
It is not easy reaching a target state where a data-driven culture pervades, data is clean and easily accessible, insight is readily available and machine learning has automated and improved decisions across the enterprise. In most cases, the journey to get there isn’t clear, or will take so long that too much will change along the way to be certain of the path.
Very few organisations have the patience to wait for “strategic investments” to pay off, and “quick wins” often prove to be unsatisfying “empty calories” which fail to build re-usable assets. This is why we believe in an iterative approach to data transformation, which delivers tangible value at each step in the process – enabling credibility to be built in conjunction with lasting capability.
Milestones for data transformation programmes should not be oriented towards technical outcomes (e.g. data loaded into warehouse, new data quality tool deployed), they should instead by oriented towards tangible business outcomes (e.g. customer complaints reduced by 10%, cross-sell conversion rate increased by 5%). To achieve these outcomes requires data to be cleansed, governed, loaded into warehouses and machine learning models built – but these technical achievements are simply the means to an end.
Join us next time when we explore our next shift and the changes we are seeing in the process space.
More from the series
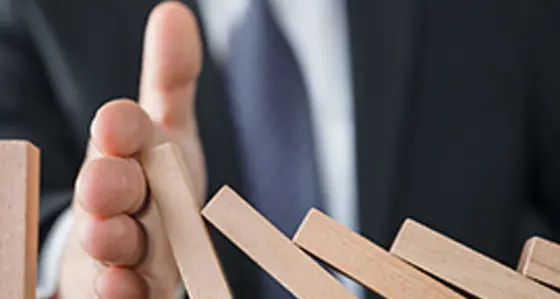
Shift 12: Organisational alignment - risk and resilience
Risk and Resilience: the shift from isolated controls and afterthought to building advocacy and trust through pro-active and integrated controls.
Read more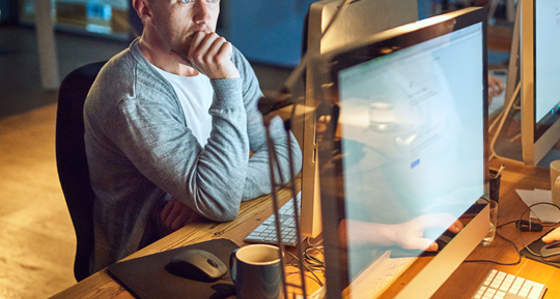
Shift 11: Organisational alignment - method
We investigate what it takes to move at the rapid pace of the market, and embrace experimentation and rapid pathfinding.
Read more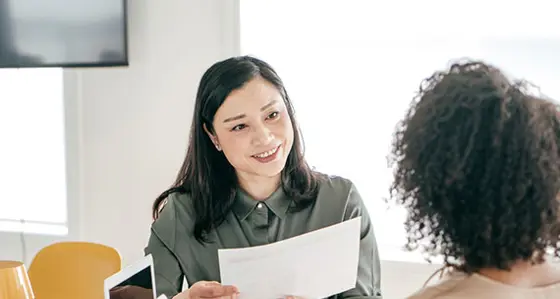
Shift 10: Organisational alignment - culture
Transforming a legacy culture isn’t always quick or easy, but it is fundamental to compete in the digital era
Read more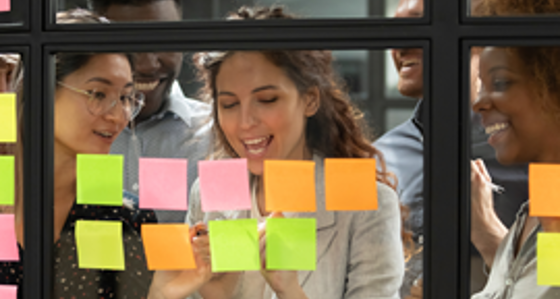
Shift 9: From large, siloed departments to small, end-to-end multidisciplinary teams
We explore the shifts that enable organisations to be more adaptable in these uncertain times.
Read more